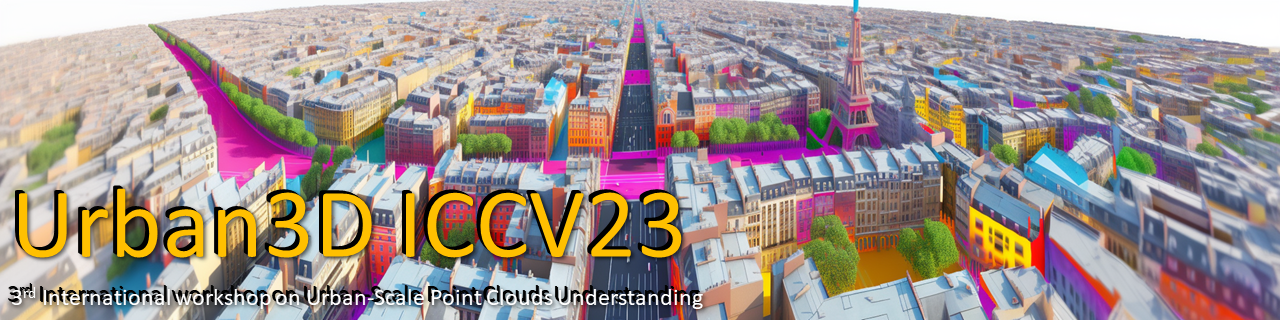
The 3D world around us is composed of a rich variety of objects: buildings, bridges, trees, cars, rivers, and so forth, each with distinct appearance, morphology, and function. Giving machines the ability to precisely segment and label these diverse objects is of key importance to allow them to interact competently within our physical world, for applications such as scene-level robot navigation, autonomous driving, and even large-scale urban 3D modeling, which is critical for the future of smart city planning and management.
Over the past years, remarkable advances in techniques for 3D point cloud understanding have greatly boosted performance. Although these approaches achieve impressive results for object recognition and semantic segmentation, almost all of them are limited to extremely small 3D point clouds, and are difficult to be directly extended to large-scale point clouds. [Bilibili Live] [YouTube Live]
The 3rd Challenge on Large Scale Point-cloud Analysis for Urban Scenes Understanding (Urban3D) at ICCV 2023 aims to establish new benchmarks for 3D semantic and instance segmentation on urban-scale point clouds. In particular, we prime the challenge with both SensatUrban and STPLS3D datasets. SensatUrban consists of large-scale subsections of multiple urban areas in the UK. With the high quality of per-point annotations and the diverse distribution of semantic categories. STPLS3D is composed of both real-world and synthetic environments which cover more than 17 km2 of the city landscape in the U.S. with up to 18 fine-grained semantic classes and 14 instance classes. These two datasets are complementary to each other and allow us to explore a number of key research problems and directions for 3D semantic and instance learning in this workshop. We aspire to highlight the challenges faced in 3D segmentation on extremely large and dense point clouds of urban environments, sparking innovation in applications such as smart cities, digital twins, autonomous vehicles, automated asset management of large national infrastructures, and intelligent construction sites. We hope that our datasets, and this workshop could inspire the community to explore the next level of 3D learning. Specifically, We encourage researchers from a wide range of background to participate in our challenge, the topics including but not limited to:
- Semantic segmentation of large-scale 3D point clouds.
- Instance segmentation of 3D point clouds.
- Weakly (self) supervised learning in 3D point clouds analysis.
- Domain adaptation of heterogeneous 3D point clouds.
- Learning from imbalanced 3D point clouds.
- 3D point cloud acquisition & visualization.
- 3D object detection & reconstruction.
Call for Contributions
Urban3D Challenges@ICCV'2023
The Urban3D Challenges are hosted on Codalab, and can be found at:
-
Track 1: 3D Semantic Segmentation of Urban-scale Point Clouds.
- Urban3D Challenge: https://codalab.lisn.upsaclay.fr/competitions/7113
- SensatUrban dataset: http://point-cloud-analysis.cs.ox.ac.uk/
- SensatUrban API: https://github.com/QingyongHu/SensatUrban
-
Track 2: 3D Instance Segmentation of Urban-scale Point Clouds.
- STPLS3D Challenge: https://codalab.lisn.upsaclay.fr/competitions/4646
- STPLS3D dataset: https://www.stpls3d.com
- STPLS3D API: https://github.com/meidachen/STPLS3D
We are thankful to our sponsor for providing the following prizes. The prize award will be granted to the Top 3 individuals and teams for Each Challenge Track on the leaderboard that provide a valid submission.
|
$1,500 USD | courtesy of ![]() |
|
$1,000 USD | courtesy of ![]() |
|
$500 USD | courtesy of ![]() |
Preliminary Program Outline
09:00-09:10 | Welcome Introduction |
09:10-09:40 | Invited Talk (Talk 1) |
09:40-10:10 | Invited Talk (Talk 2) |
10:10-10:40 | Invited Talk (Talk 3) |
11:40-11:00 | Coffee break |
11:00-11:15 | Winner Talk 1 (Track 1) |
11:15-11:30 | Winner Talk 1 (Track 2) |
11:30-11:45 | Winner Talk 2 (Track 2) |
11:45-12:00 | Closing Remarks |
Invited Keynote Speakers
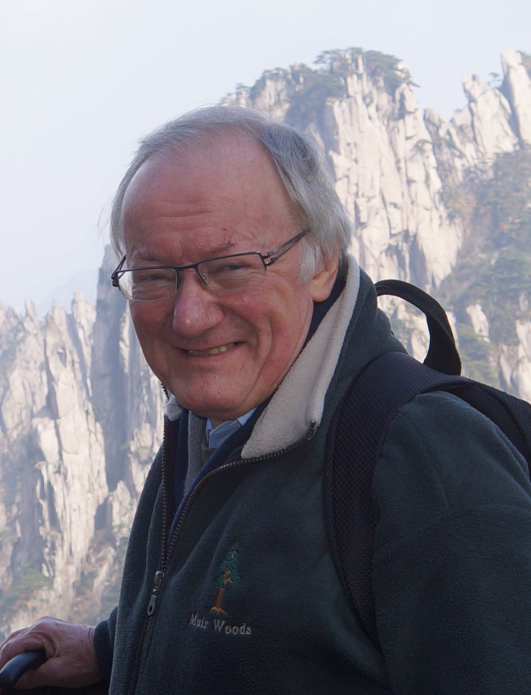
University College London
From 2D to 3D and Back Again Through Digital Twins
Michael Batty is Bartlett Professor of Planning at University College London where he is Chair of the Centre for Advanced Spatial Analysis (CASA). He has worked on computer models of cities and their visualisation since the 1970s and has published several books, such as Cities and Complexity (MIT Press, 2005) which won the Alonso Prize of the Regional Science Association in 2011, and most recently The New Science of Cities (MIT Press, 2013). His blogs www.complexcity.info cover the science underpinning the technology of cities and his posts and lectures on big data and smart cities are at www.spatialcomplexity.info . His research group is working on simulating long term structural change and dynamics in cities as well as their visualisation. Prior to his current position, he was Professor of City Planning and Dean at the University of Wales at Cardiff and then Director of the National Center for Geographic Information and Analysis at the State University of New York at Buffalo. He is a Fellow of the British Academy (FBA ), the Academy of Social Sciences (FAcSS ) and the Royal Society (FRS ), was awarded the CBE in the Queen’s Birthday Honours in 2004 and the 2013 recipient of the Lauréat Prix International de Géographie Vautrin Lud (generally known as the 'Nobel de Géographie') . This year 2015 he received the Founders Medal of the Royal Geographical Society for his work on the science of cities. In 2016 he received the Gold Medal of the Royal Town Planning Institute, and the Senior Scholars Award of the Complex Systems Society. He has Honorary Doctorates form the State University of New York and from the University of Leicester.
In the fields of architecture and planning, 3D models primarily representing the physical form of buildings and cities, came hard on the heels of computer cartography and 2D mapping. In this paper, we will review how geographic information systems rapidly acquired the ability to simulate urban form in 3D and how these ideas then moved on to incorporate various kinds of virtual reality and virtual worlds as an integral part of this representation. Currently many ideas associated with the metaverse are being developed and the notion of a 3D model as a fairly accurate portrayal of a ‘real’ building to a ‘real’ city is being rapidly relaxed. We review this transition from 2D to 3D and the ways different elements of the city, much being fashioned from data streamed in real time, are being incorporated into such models. We illustrate this evolution with our model of Virtual London (ViLo) and we then contrast this with other models that focus on London but are much more focussed on urban processes than on visual representation. In this sense, the different models focus on the same place and as such they are digital twins of one another. This paper will focus on how very different kinds of models in 2D and 3D relate to one another suggesting that in the future, the idea of many models of the same place being built – many twins of each other – will become the norm as developing such models becomes ever easier to construct and as data for their form becomes ever more available through various kinds of sensing.
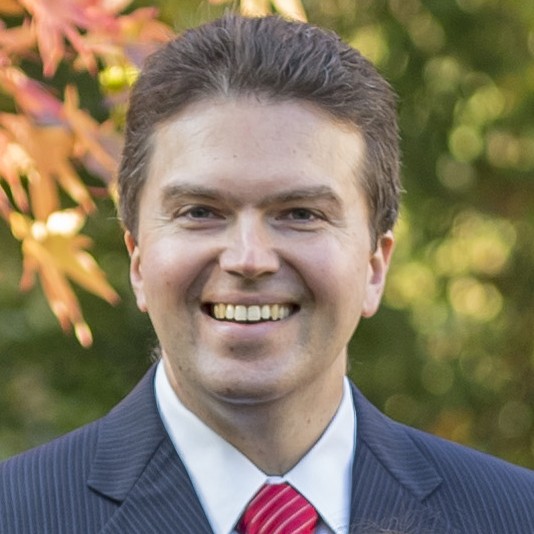
University of Cambridge
Digital Twinning the Built Environment
Prof Ioannis Brilakis is the Laing O'Rourke Professor of Civil & Information Engineering and the Director of the Construction Information Technology Laboratory at the Division of Civil Engineering of the Department of Engineering at the University of Cambridge. He completed his PhD in Civil Engineering at the University of Illinois, Urbana Champaign in 2005. He then worked as an Assistant Professor at the Departments of Civil and Environmental Engineering, University of Michigan, Ann Arbor (2005-2008) and Georgia Institute of Technology, Atlanta (2008-2012) before moving to Cambridge in 2012 as a Laing O’Rourke Lecturer. He was promoted to Reader in October 2017 and to Professor in 2021. He has also held visiting posts at the Department of Computer Science, Stanford University as a Visiting Associate Professor of Computer Vision (2014) and at the Technical University of Munich as a Visiting Professor, Leverhulme International Fellow (2018-2019), and Hans Fischer Senior Fellow (2019-2023). He is a recipient of the 2022 EC3 Scherer Award, 2022 EC3 Thorpe Medal, 2019 ASCE J. James R. Croes Medal, the 2018 ASCE John O. Bickel Award, the 2013 ASCE Collingwood Prize, the 2012 Georgia Tech Outreach Award, a 2010 NSF CAREER award, and a 2009 ASCE Associate Editor Award. Dr Brilakis is an author of over 200 papers in peer-reviewed journals and conference proceedings, an Associate Editor of the ASCE Computing in Civil Engineering, ASCE Construction Engineering and Management, Elsevier Automation in Construction, and Elsevier Advanced Engineering Informatics Journals, and the lead founder of the European Council on Computing in Construction.
Digital Twinning methods can produce a reliable digital record of the built environment and enable owners to reliably protect, monitor and maintain the condition of their asset. The built environment is comprised of large assets that need significant resource investments to design, construct, maintain and operate them. Improving productivity, i.e., efficiency and effectiveness, and creating new, disruptive ways to address existing problems throughout their lifecycle can generate significant performance improvements in cost, time, quality, safety, sustainability, and resilience metrics for all involved parties. Creating and maintaining an up-to-date electronic record of built environment assets in the form of rich Digital Twins can help generate such improvements. This talk introduces research conducted at the University of Cambridge on inexpensive methods for generating object-oriented infrastructure geometry, detecting, and mapping visible defects on the resulting Digital Twin, automatically extracting defect spatial measurements, and sensor and sensor data modelling. The results of these methods are further exploited through their application in design for manufacturing and assembly (DfMA), mixed-reality-enabled mobile inspection, and proactive asset protection from accidental damage.
Towards Global 3D/4D Urban Modeling from Space
Xiao Xiang Zhu is the Chair Professor of Data Science with Earth Observation, Technical University of Munich, and was the Founding Head of the Department “EO Data Science,” Remote Sensing Technology Institute, German Aerospace Center (DLR), Cologne, Germany. She received the master’s (M.Sc.) degree, doctor of engineering (Dr.-Ing.) degree, and “Habilitation” degree in signal processing from Technical University of Munich (TUM), Munich, Germany, in 2008, 2011, and 2013, respectively. She is also the IEEE Fellow. Since 2019, she has been a Co-Coordinator of the Munich Data Science Research School, Munich, and has been the Head of the Helmholtz Artificial Intelligence—Research Field Aeronautics, Space and Transport, Munich. Since 2020, she has been the Director of the International Future Artificial Intelligence (AI) Lab—Artificial Intelligence for Earth Observation (AI4EO): Reasoning, Uncertainties, Ethics and Beyond, Munich. Since 2020, she has also been the Co-Director of the Munich Data Science Institute (MDSI), TUM. Her research interests include remote sensing and Earth observation, signal processing, machine learning, and data science, with their applications in tackling societal grand challenges, e.g., global urbanization, Union Nations’ Sustainable Development Goals (UN’s SDGs), and climate change.
Geoinformation derived from Earth observation satellite data is indispensable for many scientific, governmental and planning tasks. Geoscience, environmental sciences, sustainable development, resource management, civil security, disaster relief, as well as planning and decision support are just a few examples. This talk will present a series of novel algorithms, both model-based and data-driven, aiming at delivering the world's first global 3D and 4D urban model using earth observation satellite data from multiple sensors.
Awards
Track 1: 3D Semantic Segmentation of Urban-scale Point Clouds.
- 1st Place:   Dimensionality
Jiacheng Deng, Xinjun Li, Jiahao Lu, and Tianzhu Zhang
- 1st Place:   USTC-IAT-United
Jun Yu, Ruiyu Liu, Zhen Kan, Gongpeng Zhao, Renda Li, Renjie Lu, Bingyuan Zhang, Shuoping Yang, Leilei Wang, Zhenchao Ouyang - 2nd Place:   PEACE
Ye Runchun, Lin Yin - 3rd Place:   NJUST-404
Zhipeng Zhou, Kailong Xu, Tianyang Qiu, Kelong Sheng
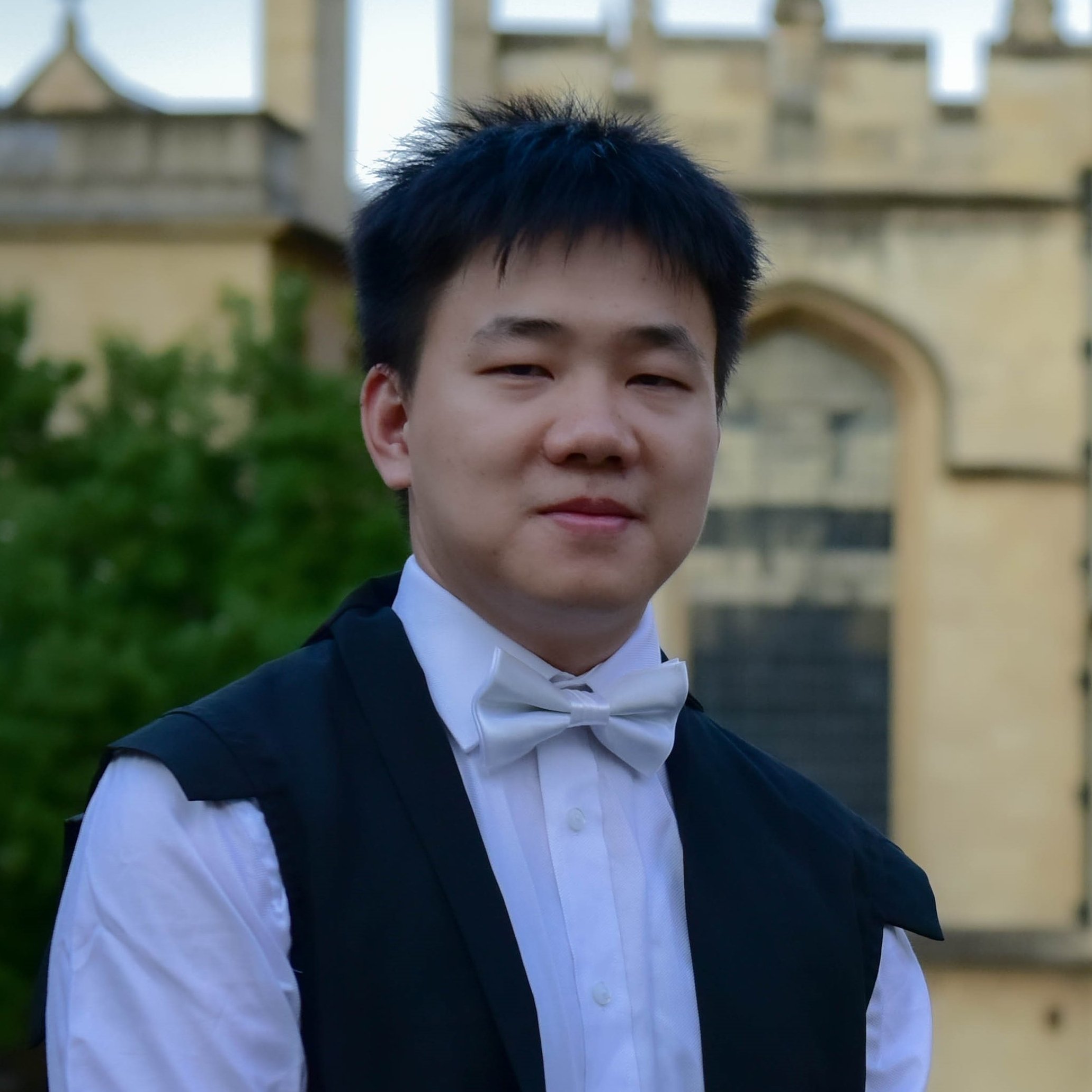
University of Oxford
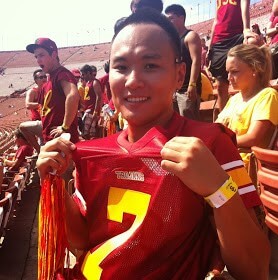
University of Southern California - Institute for Creative Technologies
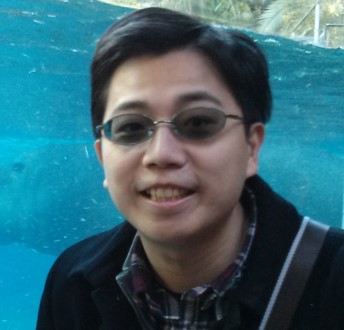
University of Southern California - Institute for Creative Technologies
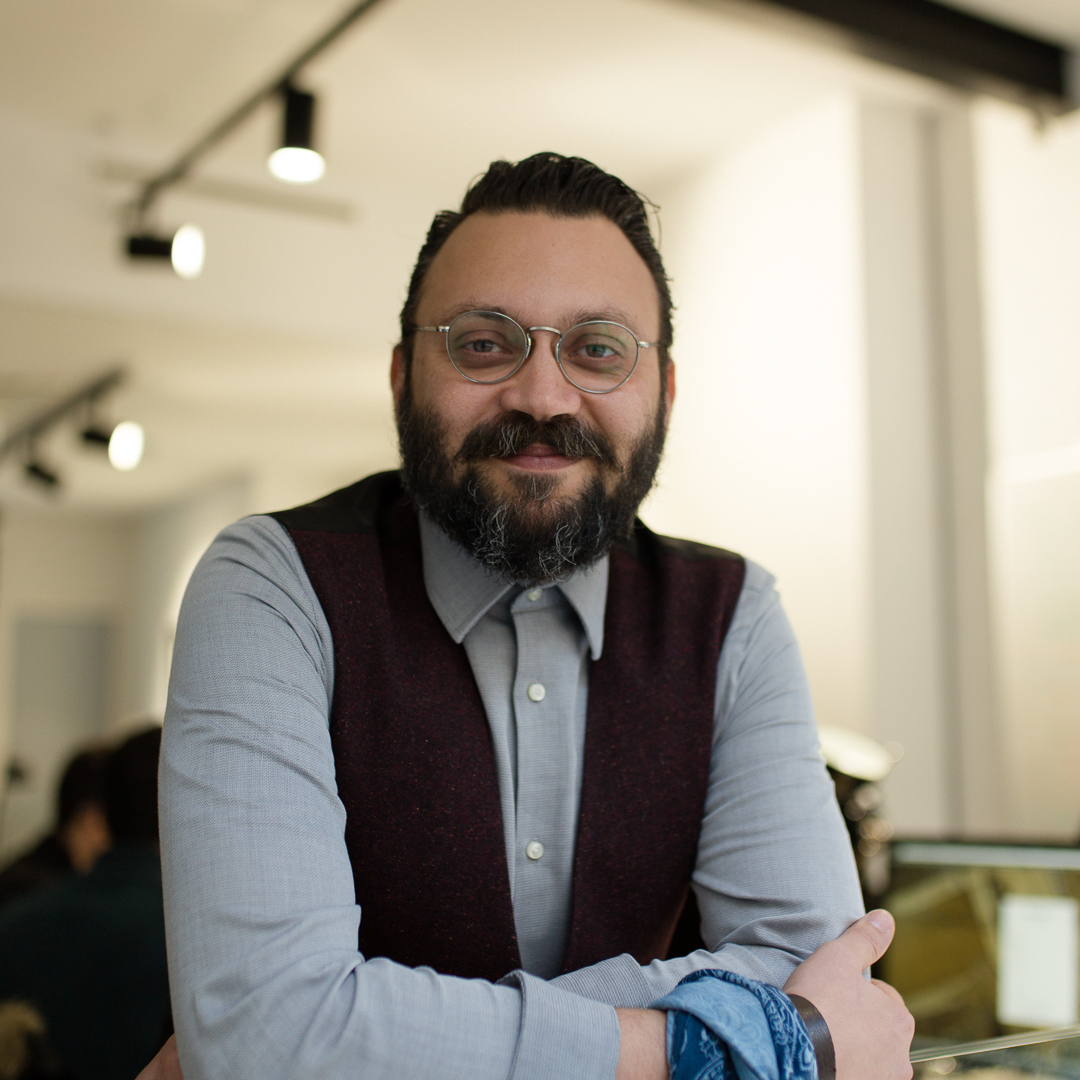
Sensat LTD.
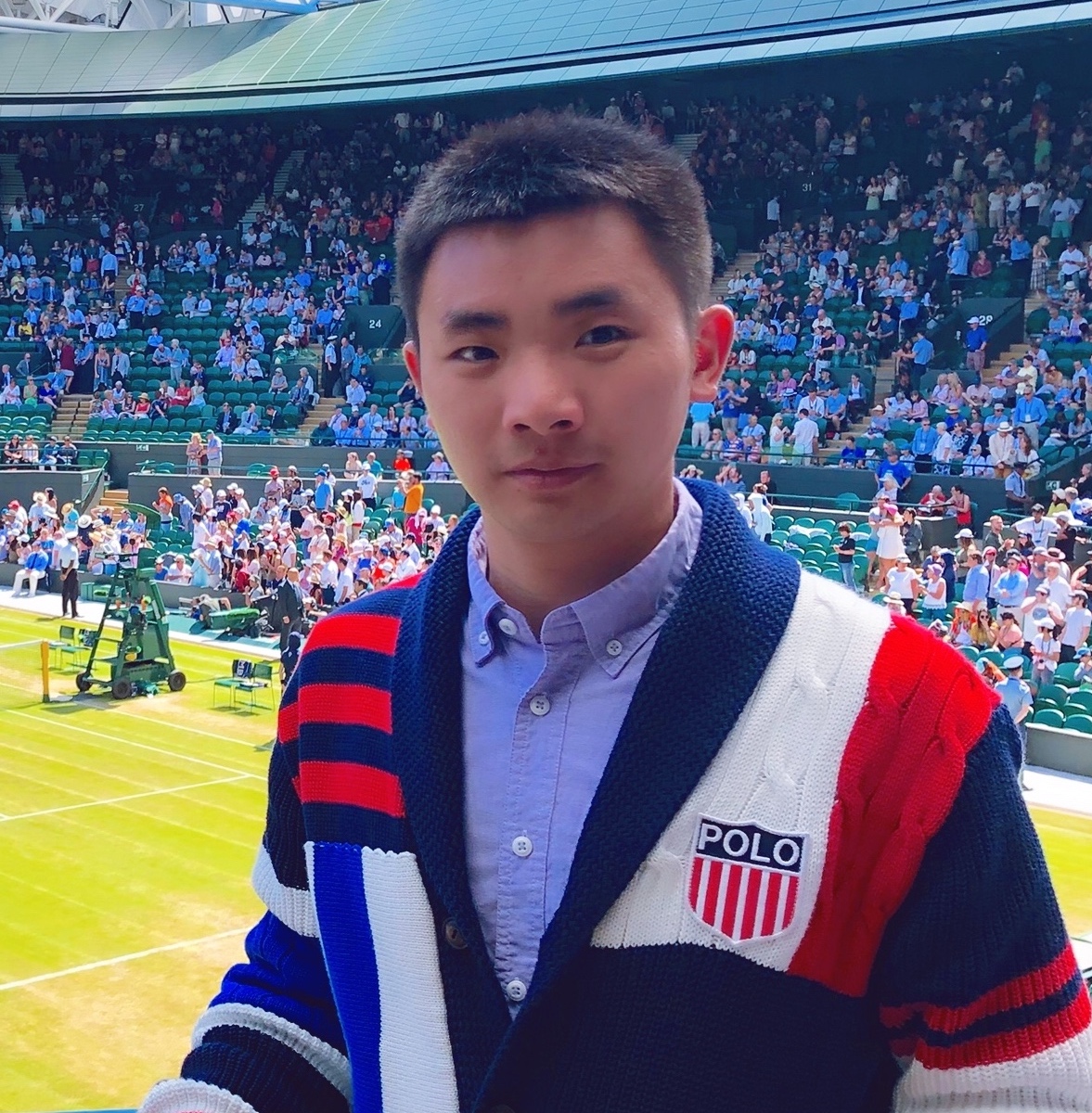
The Hong Kong Polytechnic University
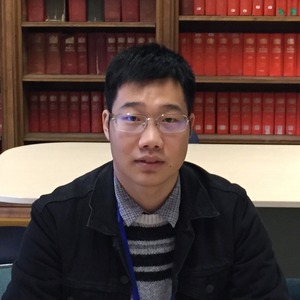
The Hong Kong Polytechnic University
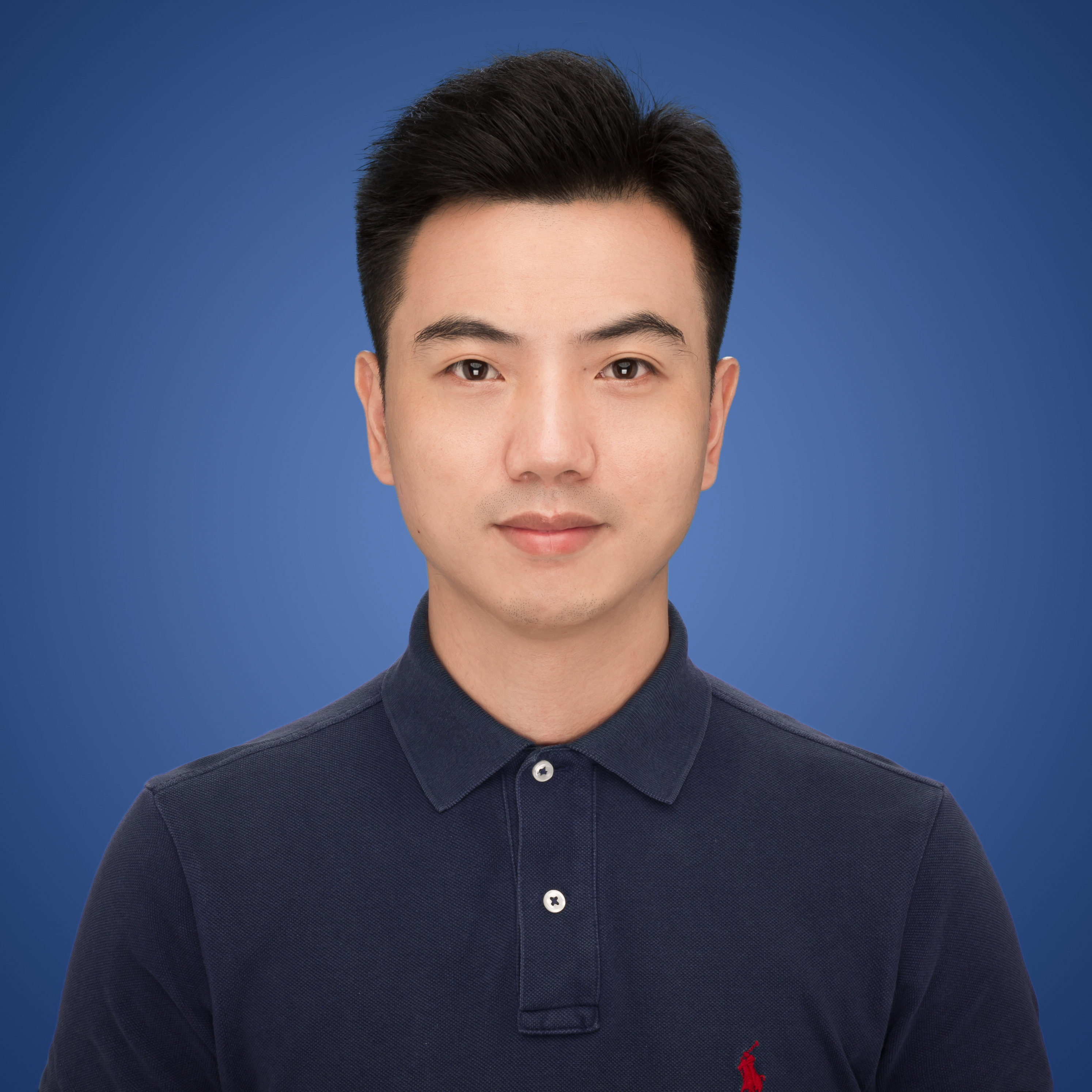
National University of Defense Technology
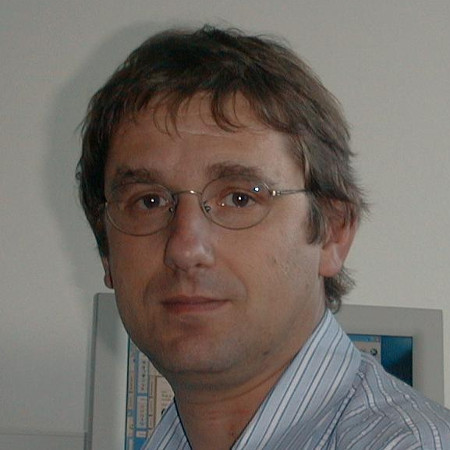
University of Birmingham
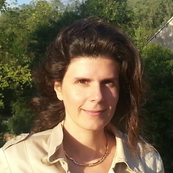
University of Oxford
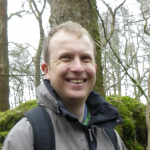
University of Oxford
- Urban3D@ICCV2021: https://urban3dchallenge.github.io/2021 [Replay]
- Urban3D@ECCV2022: https://urban3dchallenge.github.io/2022 [Replay]